Selecting the right dataset for your AI projects doesn’t need to be a chore. As AI professionals, we know that the quality of our datasets plays a crucial role in the success of our machine-learning projects. Low-quality or poorly curated datasets can lead to problems such as bias, error, and irrelevant results, while high-quality datasets can lead to more accurate and reliable models.
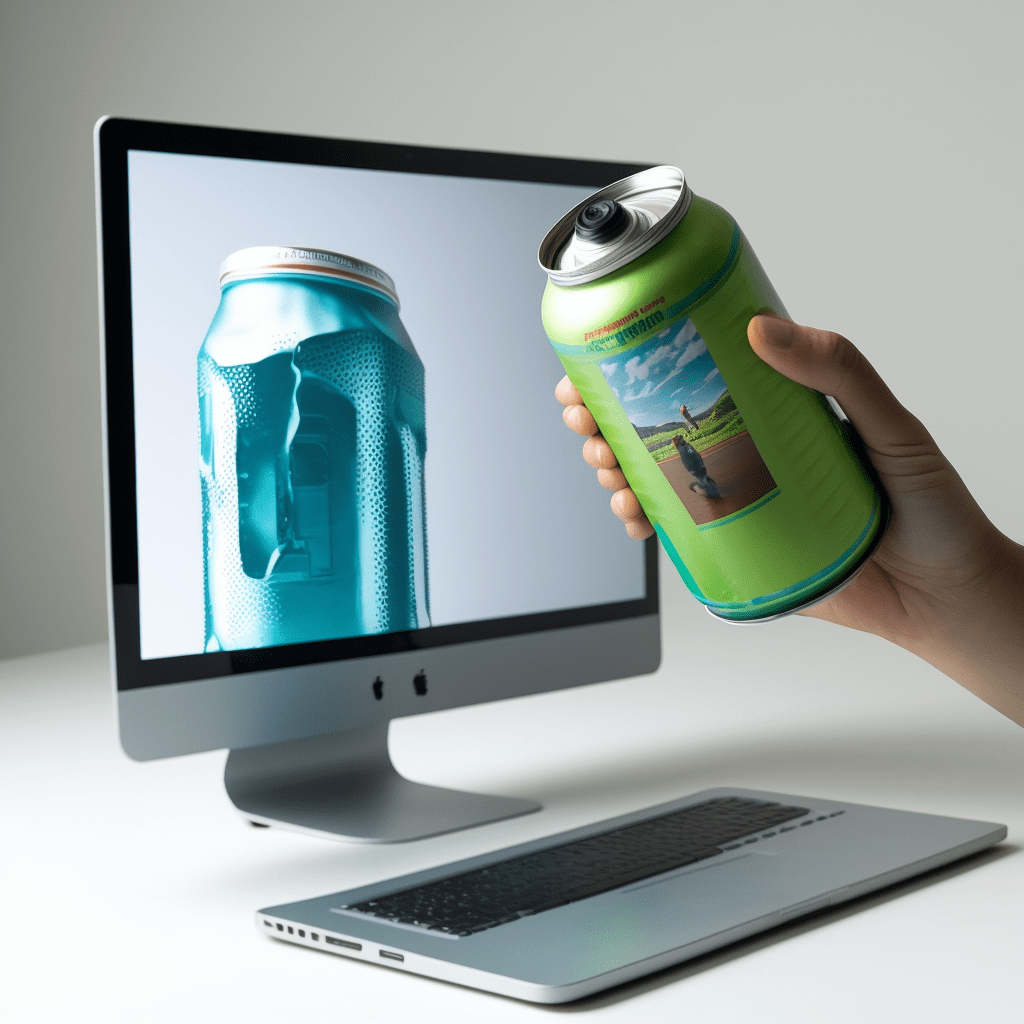
But with so many datasets available online, how do you choose the right one for your project? Here are some tips and guidelines to help you find and select the best dataset for your needs:
- Look for datasets that are large and diverse: Machine learning algorithms benefit from exposure to a wide range of data, so look for datasets that are large and diverse. This will help the model learn more about the underlying patterns and relationships in the data, and improve its ability to generalize to new situations.
- Check for data quality and relevance: Before using a dataset, make sure to evaluate its quality and relevance. Is the data complete and accurate? Is it relevant to your specific task or domain? If the data is flawed or unrelated to your goals, it will likely hurt the performance of your model.
- Consider domain expertise: If you are working on a specific task or in a particular domain, it can be helpful to use datasets that have been curated or annotated by experts in that field. This can help ensure that the data is relevant and meaningful for your specific goals.
- Explore preprocessing options: Depending on your needs, you may also want to consider preprocessing options to get the most out of your dataset. This might include cleaning and normalizing the data, selecting relevant features, or applying other types of data transformations to improve the model’s performance.
To illustrate the practical applications and benefits of following these tips, let’s look at a couple of success stories. In one case study, a team of researchers used a large and diverse dataset to train a machine learning model to accurately predict the likelihood of a patient developing a particular disease. The model achieved impressive results, outperforming traditional methods by a significant margin. In another case study, a company used a dataset with expert-curated annotations to train a machine learning model to classify and categorize products. The model was able to accurately and efficiently categorize over a million products, saving the company significant time and resources.
As these examples show, selecting the right dataset for your AI projects is essential for the success of your AI projects. By following the tips and guidelines outlined above, you can increase the chances of success and achieve better results.
For more resources and tools to help you find and evaluate datasets for your machine learning projects, check out the following links: